
Users often need to integrate information from multiple data sources. AutoD proposes autonomous systems that without any or with minimal expert intervention and progressively discovers and integrates relevant information from multiple data sources. It leverages end users' feedback to learn how to retrieve information relevant to each entity in a dataset from external data sources.
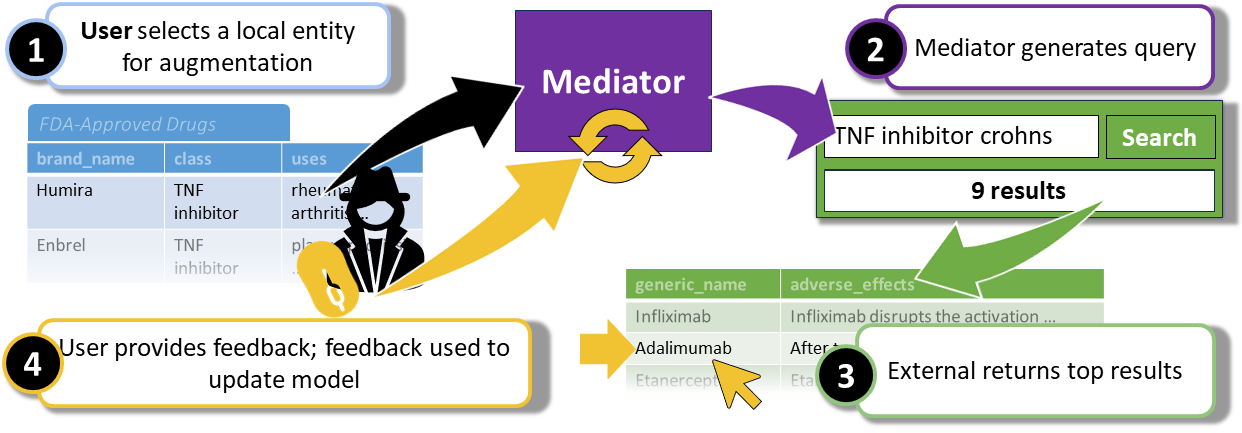
Publications
-
Generating Data Integration Queries Using Large Language Models [Slides] [Code+Data] [BibTeX]
Christopher Buss, Jasmin Mousavi, Mikhail Tokarev, Arash Termehchy, David Maier, and Stefan Lee
The Proceedings of VLDB Workshop on Databases and Large Language Models (LLMDB), August, 2023.
Also presented at the 2nd NeurIPS Table Representation Learning Workshop, December, 2023.
-
Effective Entity Augmentation By Querying External Data Sources [Slides] [Video] [Code+Data] [BibTeX]
Christopher Buss, Jasmin Mousavi, Mikhail Tokarev, Arash Termehchy, David Maier, and Stefan Lee
The Proceedings of VLDB Endowment (PVLDB), Vol16, 2023.
-
Progressive Interaction for Autonomous Entity Matching [Slides]
Ben McCamish, Arash Termehchy
The Proceedings VLDB Workshop on Polystore Systems (Poly), September 2018.
People
-
Arash Termehchy
-
Christopher Buss
-
Jasmin Mousavi
-
Mahdis Safari
-
Mikhail Tokarev